This course covers practical algorithms and the theory for machine learning from a variety of perspectives. Topics include supervised learning (generative, discriminative learning, parametric, non-parametric learning, deep neural networks, support vector Machines), unsupervised learning (clustering, dimensionality reduction, kernel methods). The course will also discuss recent applications of machine learning, such as computer vision, data mining, natural language processing, speech recognition and robotics. Students will learn the implementation of selected machine learning algorithms via python and PyTorch.

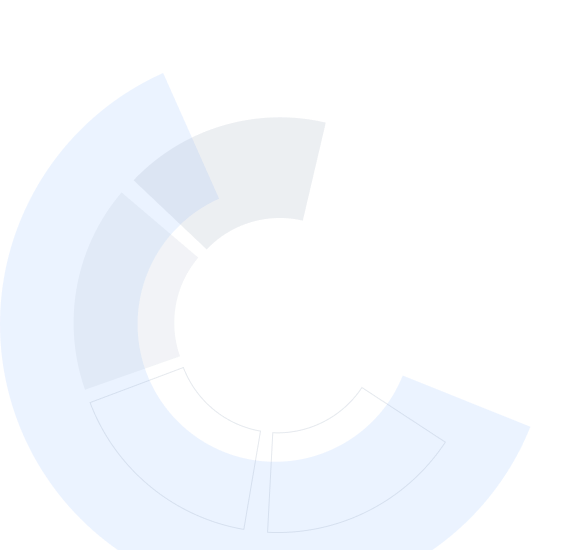
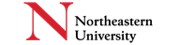
Wichtige Details

Zu Ihrem LinkedIn-Profil hinzufügen
Januar 2025
7 Aufgaben
Erfahren Sie, wie Mitarbeiter führender Unternehmen gefragte Kompetenzen erwerben.
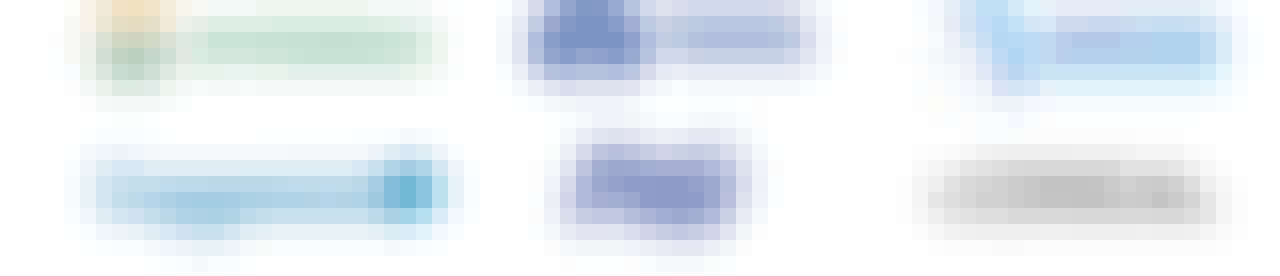
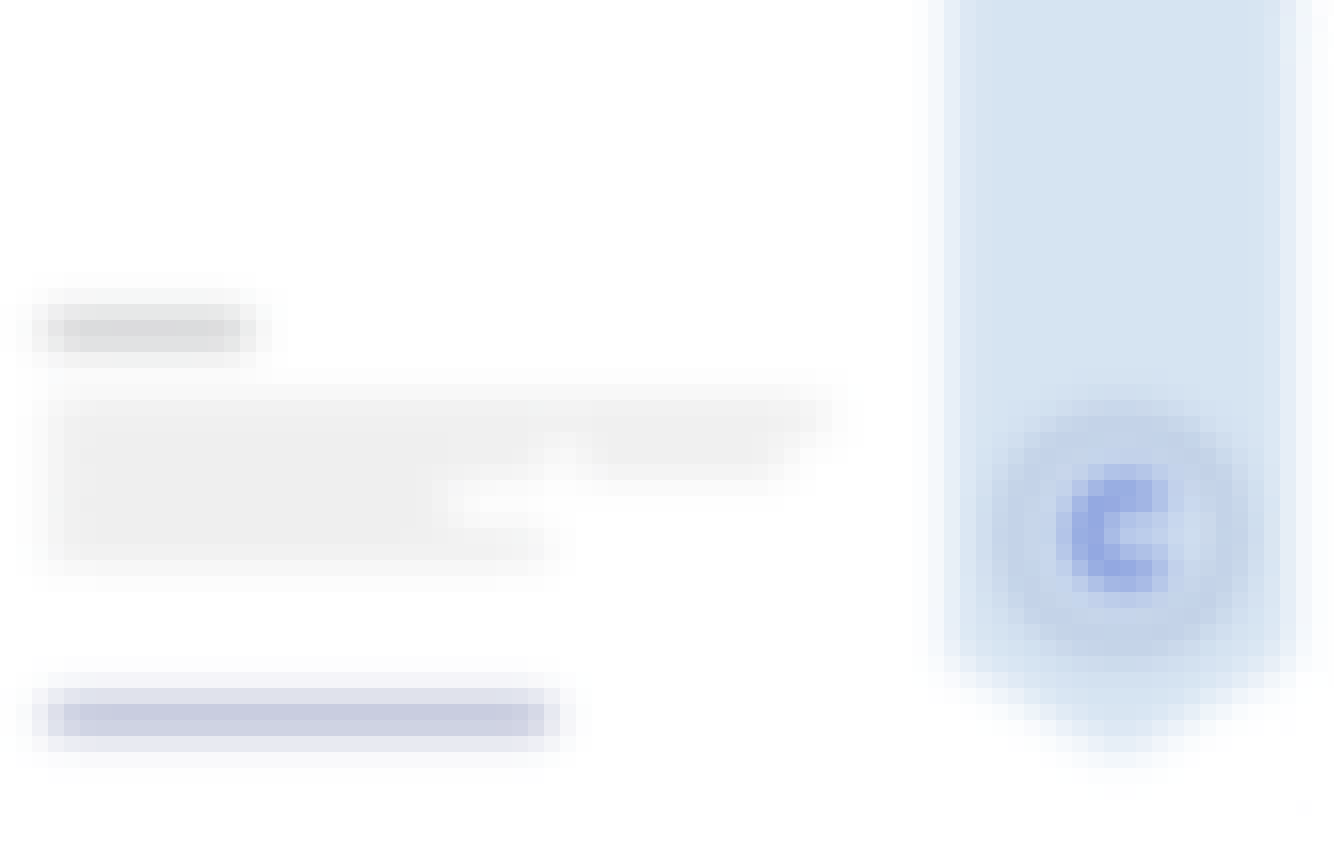
Erwerben Sie ein Karrierezertifikat.
Fügen Sie diese Qualifikation zur Ihrem LinkedIn-Profil oder Ihrem Lebenslauf hinzu.
Teilen Sie es in den sozialen Medien und in Ihrer Leistungsbeurteilung.
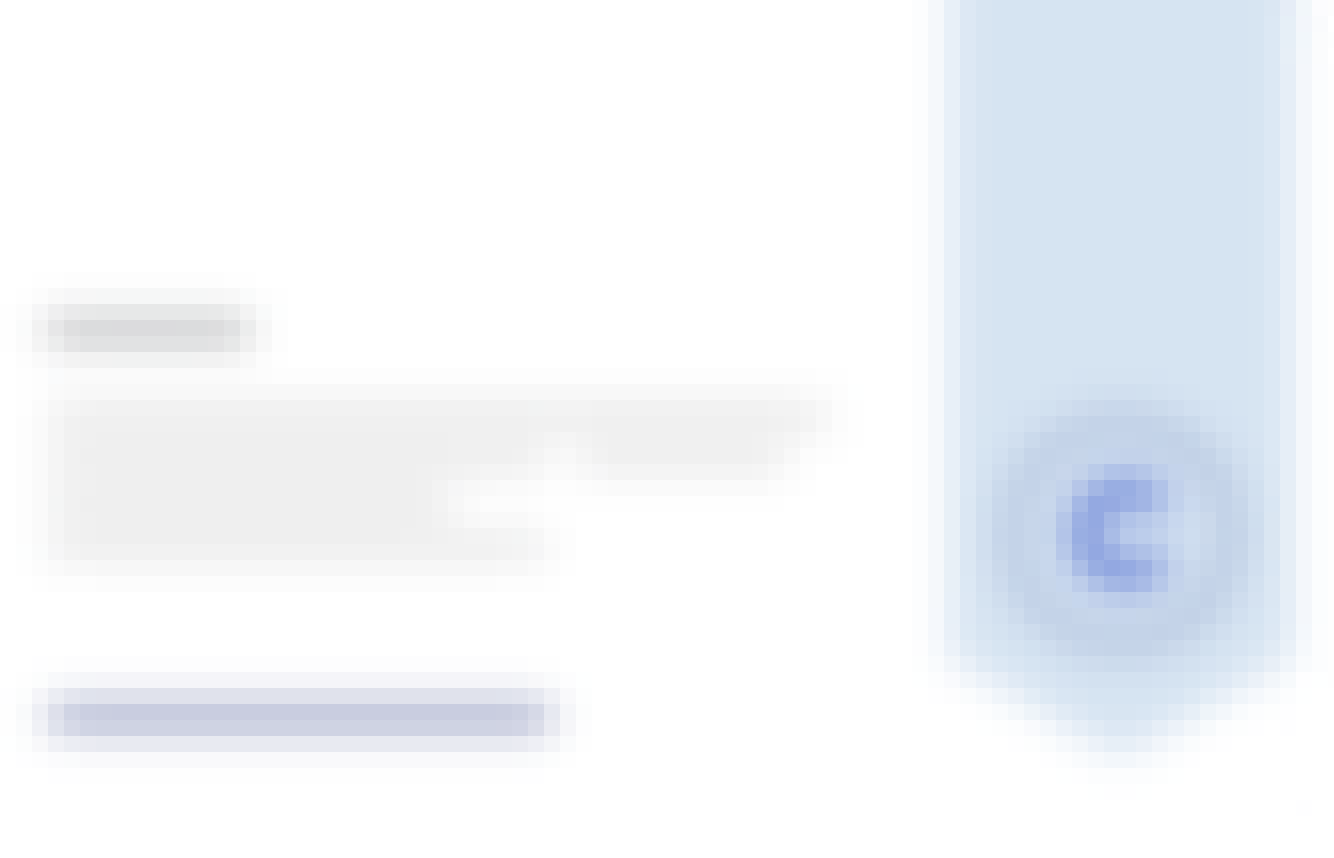
In diesem Kurs gibt es 4 Module
This week provides an introduction to the field of statistical learning, exploring its scope and practical applications across various domains. Students will analyze how statistical learning techniques are used to make predictions, infer relationships, and uncover patterns in complex datasets. The module also offers a review of the key concepts essential for success in the course, including statistical models, data handling, and learning algorithms. By the end of the module, you will have a solid understanding of statistical learning principles and be prepared to apply them in real-world scenarios, laying the foundation for deeper exploration in machine learning and data science.
Das ist alles enthalten
1 Video7 Lektüren1 Aufgabe1 Diskussionsthema
This week introduces you to the concept of Maximum Likelihood Estimation (MLE) and its application in statistical modeling. You will gain a thorough understanding of how to mathematically implement MLE and apply it to real-world datasets. The week will revisit foundational concepts of convex optimization, offering a solid foundation in optimization techniques. Additionally, the iterative process of the gradient descent algorithm will be explored, allowing you to understand and implement this method for finding optimal solutions in machine learning models. Through a combination of theoretical knowledge and practical application, you will build essential skills in statistical estimation and optimization, preparing for advanced studies in machine learning and data analysis.
Das ist alles enthalten
2 Videos3 Lektüren2 Aufgaben2 Diskussionsthemen
In this module, you will gain a comprehensive understanding of supervised machine learning from model training to evaluation. You’ll interpret each step in the learning process and apply training and evaluation techniques to real-world data. This will enable you to fit and assess models, while addressing issues like overfitting and underfitting. By exploring the bias-variance trade-off, you can optimize models for greater accuracy and reliability. Cross-validation methods are also covered, equipping students with robust tools for model assessment and performance analysis. This week will combine theoretical insights preparing you for the advanced work in machine learning.
Das ist alles enthalten
2 Videos4 Lektüren2 Aufgaben
This module, we will focus on the foundational principles of linear regression, a key technique in predictive modeling. You will learn to apply linear regression models and derive the ordinary least squares (OLS) formulation, gaining insight into how OLS is used to fit data accurately. We will also cover solution methods, including gradient descent and convex optimization, which provides a toolkit for efficient model training. You will explore regularization techniques to enhance model robustness and prevent overfitting. By implementing these regularized regression models in Python, you will gain hands-on experience in model optimization.
Das ist alles enthalten
2 Videos2 Lektüren2 Aufgaben1 Diskussionsthema
Dozent

Empfohlen, wenn Sie sich für Algorithms interessieren
Politecnico di Milano
Johns Hopkins University
The University of Chicago
University of Washington
Warum entscheiden sich Menschen für Coursera für ihre Karriere?





Neue Karrieremöglichkeiten mit Coursera Plus
Unbegrenzter Zugang zu 10,000+ Weltklasse-Kursen, praktischen Projekten und berufsqualifizierenden Zertifikatsprogrammen - alles in Ihrem Abonnement enthalten
Bringen Sie Ihre Karriere mit einem Online-Abschluss voran.
Erwerben Sie einen Abschluss von erstklassigen Universitäten – 100 % online
Schließen Sie sich mehr als 3.400 Unternehmen in aller Welt an, die sich für Coursera for Business entschieden haben.
Schulen Sie Ihre Mitarbeiter*innen, um sich in der digitalen Wirtschaft zu behaupten.
Häufig gestellte Fragen
Access to lectures and assignments depends on your type of enrollment. If you take a course in audit mode, you will be able to see most course materials for free. To access graded assignments and to earn a Certificate, you will need to purchase the Certificate experience, during or after your audit. If you don't see the audit option:
The course may not offer an audit option. You can try a Free Trial instead, or apply for Financial Aid.
The course may offer 'Full Course, No Certificate' instead. This option lets you see all course materials, submit required assessments, and get a final grade. This also means that you will not be able to purchase a Certificate experience.
When you purchase a Certificate you get access to all course materials, including graded assignments. Upon completing the course, your electronic Certificate will be added to your Accomplishments page - from there, you can print your Certificate or add it to your LinkedIn profile. If you only want to read and view the course content, you can audit the course for free.
You will be eligible for a full refund until two weeks after your payment date, or (for courses that have just launched) until two weeks after the first session of the course begins, whichever is later. You cannot receive a refund once you’ve earned a Course Certificate, even if you complete the course within the two-week refund period. See our full refund policy.
Weitere Fragen
Finanzielle Unterstützung verfügbar,