This course delves into both the theoretical aspects and practical applications of data mining within the field of engineering. It provides a comprehensive review of the essential fundamentals and central concepts underpinning data mining. Additionally, it introduces pivotal data mining methodologies and offers a guide to executing these techniques through various algorithms. Students will be introduced to a range of data mining techniques, such as data preprocessing, the extraction of association rules, classification, prediction, clustering, and the exploration of complex data, and will implement a capstone project exploring the same. Additionally, we will use case studies to explore the application of data mining across diverse sectors, including but not limited to manufacturing, healthcare, medicine, business, and various service industries.

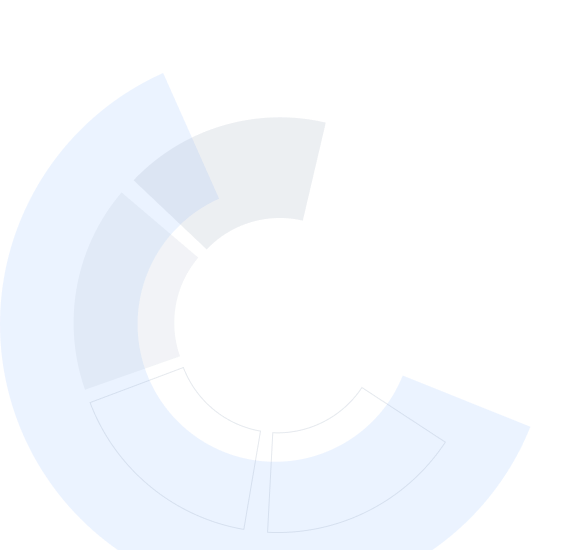
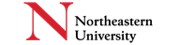
Détails à connaître

Ajouter à votre profil LinkedIn
janvier 2025
5 devoirs
Découvrez comment les employés des entreprises prestigieuses maîtrisent des compétences recherchées
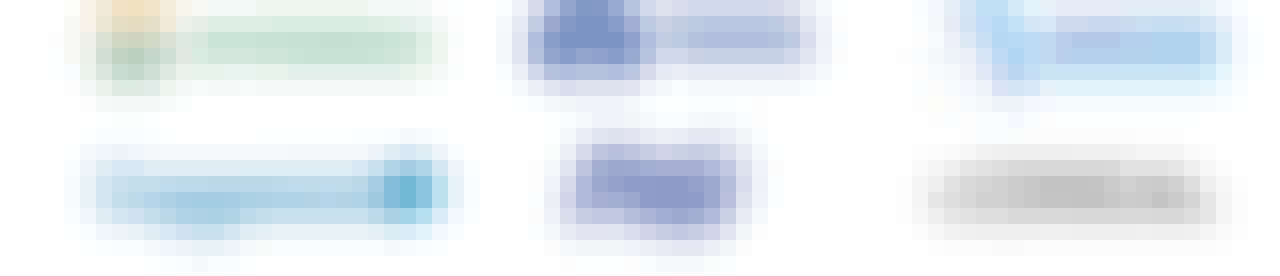
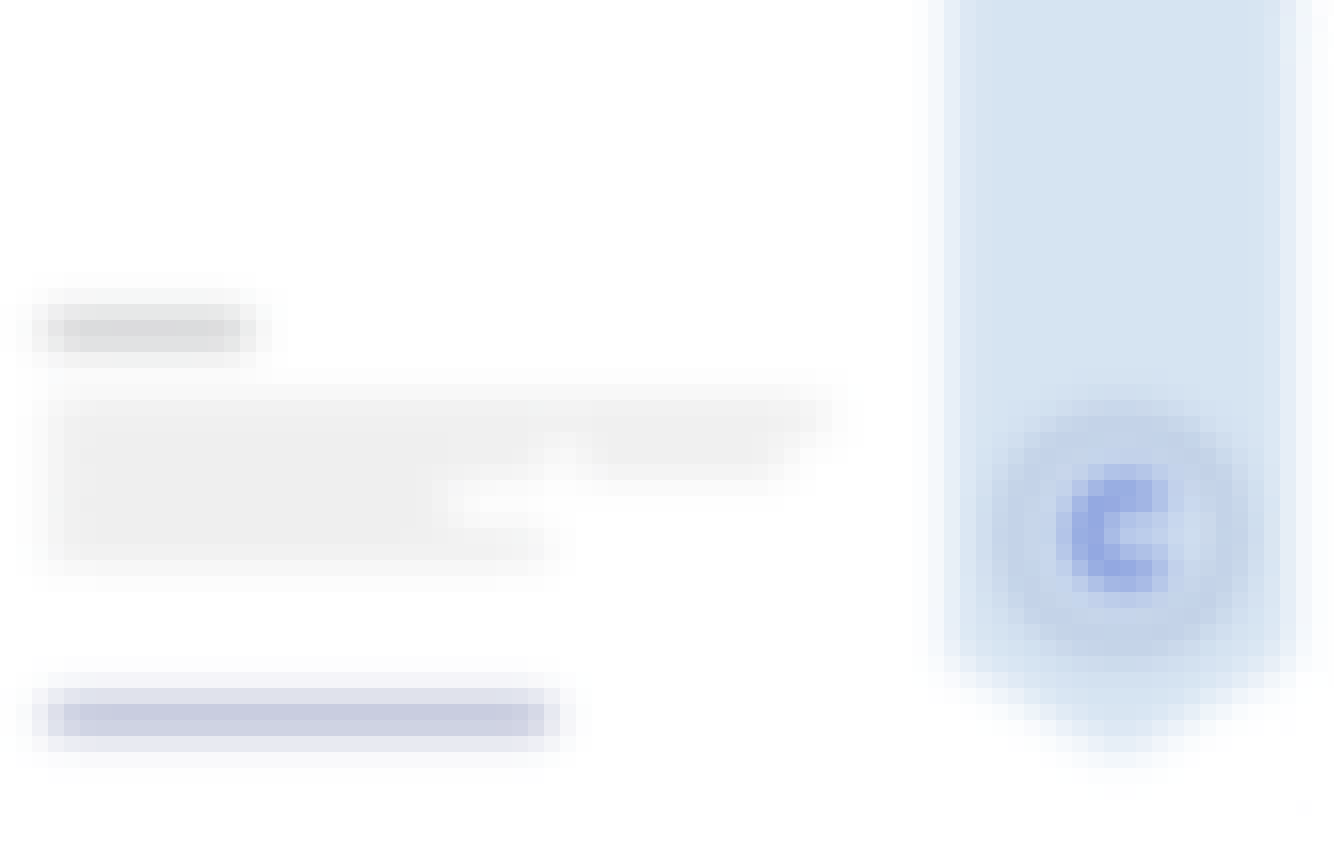
Obtenez un certificat professionnel
Ajoutez cette qualification à votre profil LinkedIn ou à votre CV
Partagez-le sur les réseaux sociaux et dans votre évaluation de performance
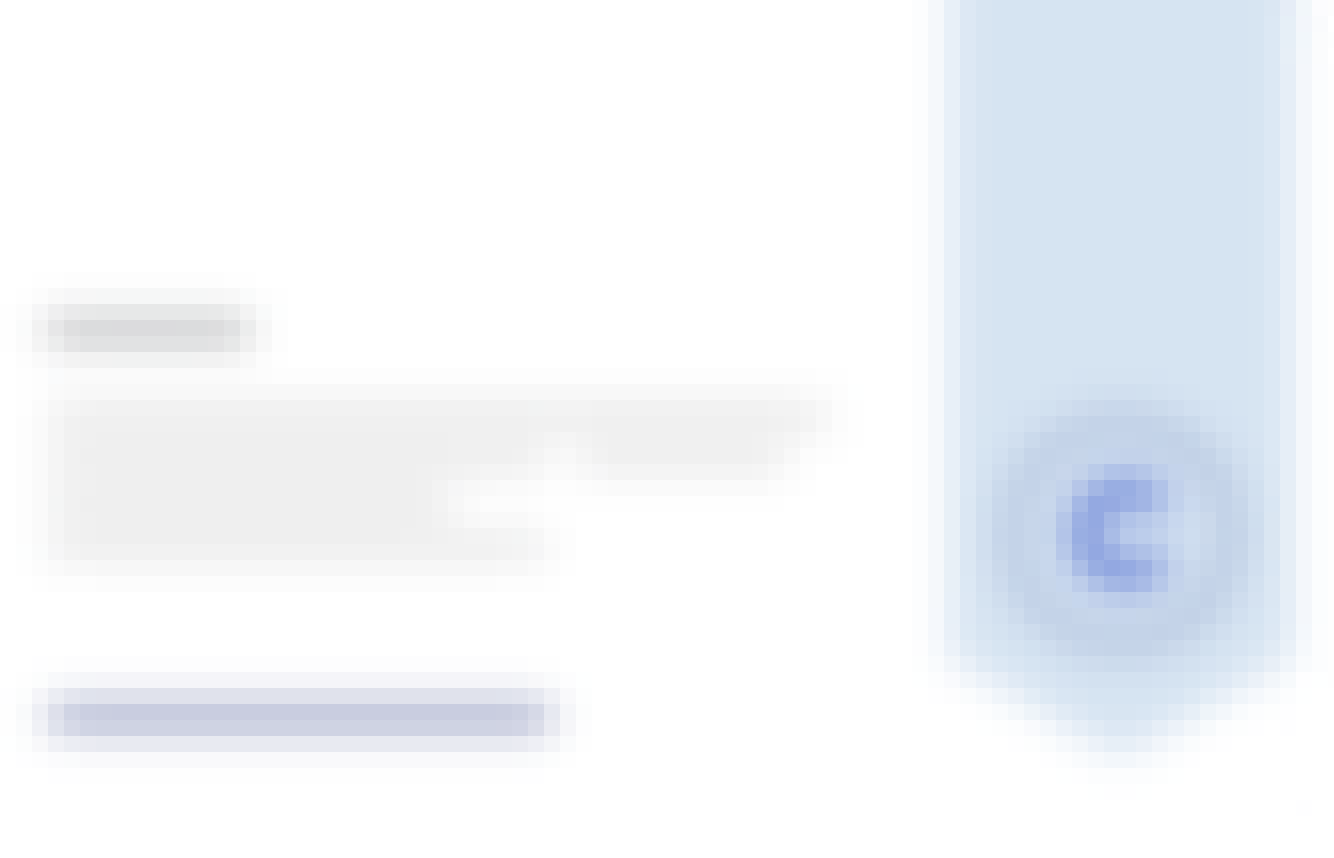
Il y a 4 modules dans ce cours
In this module, participants will explore essential data concepts across domains, understanding diverse data types, attributes, and features. They will grasp the fundamental principles, methodologies, and scope of data mining, enabling them to effectively analyze data and extract valuable insights. Through this comprehensive approach, learners will gain proficiency in utilizing key data concepts, facilitating informed decision-making and innovation across various domains.
Inclus
5 vidéos8 lectures2 devoirs2 sujets de discussion
This module aims to impart a comprehensive understanding of data concepts, spanning various domains. Participants will learn to differentiate between different data types, attributes, and features. They will explore fundamental principles and methodologies of data mining, enabling them to extract meaningful insights from datasets. By mastering these objectives, learners will be equipped with the knowledge and skills necessary to analyze data effectively and make informed decisions in diverse professional settings.
Inclus
3 vidéos13 lectures1 devoir1 sujet de discussion
Throughout this module, we will jump into the realm of dimensionality reduction, a technique for simplifying complex datasets to facilitate efficient analysis and visualization. By implementing dimensionality reduction methods such as Principal Component Analysis (PCA) and t-Distributed Stochastic Neighbor Embedding (t-SNE), we will gain insight into how to effectively reduce the number of features while preserving essential information. We'll learn to select and apply the most suitable dimensionality reduction techniques based on data types and analytical goals, thereby enhancing model performance and interpretability. This module shares the tools to navigate and extract meaningful insights from high-dimensional datasets, paving the way for more effective data analysis and decision-making.
Inclus
4 vidéos9 lectures1 devoir1 sujet de discussion
In this module, we learn the concept of the Bias-Variance Trade-off in machine learning. Striving for models that generalize well requires navigating the delicate balance between bias and variance to avoid underfitting and overfitting. Bias represents the error from oversimplifying a complex problem, while variance quantifies the model's sensitivity to different training data subsets. We explore strategies to combat bias and variance in developing models that strike the right balance between accuracy and generalization. Transitioning to regression metrics, we look at practical tools used to measure and evaluate model performance in regression tasks, focusing on metrics like Root Mean Squared Error (RMSE). Finally, we navigate the landscape of assessing model performance in binary classification tasks, exploring advanced measures like the F1-Score, Matthews Correlation Coefficient (MCC), propensity scores, and the AUC-ROC curve.
Inclus
5 vidéos10 lectures1 devoir1 sujet de discussion
Instructeur

Offert par
Recommandé si vous êtes intéressé(e) par Data Analysis
Georgia Institute of Technology
Duke University
Politecnico di Milano
Préparer un diplôme
Ce site cours fait partie du (des) programme(s) diplômant(s) suivant(s) proposé(s) par Northeastern University . Si vous êtes admis et que vous vous inscrivez, les cours que vous avez suivis peuvent compter pour l'apprentissage de votre diplôme et vos progrès peuvent être transférés avec vous.¹
Pour quelles raisons les étudiants sur Coursera nous choisissent-ils pour leur carrière ?





Ouvrez de nouvelles portes avec Coursera Plus
Accès illimité à 10,000+ cours de niveau international, projets pratiques et programmes de certification prêts à l'emploi - tous inclus dans votre abonnement.
Faites progresser votre carrière avec un diplôme en ligne
Obtenez un diplôme auprès d’universités de renommée mondiale - 100 % en ligne
Rejoignez plus de 3 400 entreprises mondiales qui ont choisi Coursera pour les affaires
Améliorez les compétences de vos employés pour exceller dans l’économie numérique
Foire Aux Questions
Access to lectures and assignments depends on your type of enrollment. If you take a course in audit mode, you will be able to see most course materials for free. To access graded assignments and to earn a Certificate, you will need to purchase the Certificate experience, during or after your audit. If you don't see the audit option:
The course may not offer an audit option. You can try a Free Trial instead, or apply for Financial Aid.
The course may offer 'Full Course, No Certificate' instead. This option lets you see all course materials, submit required assessments, and get a final grade. This also means that you will not be able to purchase a Certificate experience.
When you purchase a Certificate you get access to all course materials, including graded assignments. Upon completing the course, your electronic Certificate will be added to your Accomplishments page - from there, you can print your Certificate or add it to your LinkedIn profile. If you only want to read and view the course content, you can audit the course for free.
You will be eligible for a full refund until two weeks after your payment date, or (for courses that have just launched) until two weeks after the first session of the course begins, whichever is later. You cannot receive a refund once you’ve earned a Course Certificate, even if you complete the course within the two-week refund period. See our full refund policy.